Organisations constantly seek ways to efficiently store, manage, and analyse vast amounts of data to benefit their ability to make the right decisions quickly. Two primary solutions have emerged to address these needs: data lakes and data warehouses.
INTRODUCTION
While both serve the purpose of data storage and management, they cater to different use cases and offer distinct advantages. We'd like to provide IT leaders, data scientists, and business intelligence (BI) professionals with a comprehensive understanding of data lakes and data warehouses, offering actionable insights to help you make informed decisions.
DATA LAKES
Understanding Data Lakes
Definition And Purpose
A data lake is a centralised repository designed to store, process, and secure large amounts of:
- structured,
- semi-structured and
- unstructured data.
Unlike traditional data storage systems, data lakes allow you to store data in its raw form, providing flexibility for various analytics and machine learning applications.
Data Types And Storage
Data lakes can handle various data types, including files like:
- XML,
- JSON,
- PDFs and
- DOCs and traditional data formats.
They support multiple data sources, such as:
RDBMS, Flat files, Third-party data, Legacy Systems and Cloud data stores
Use Cases
Data lakes are particularly useful for big data analytics, machine learning, and data science. They provide a flexible data exploration and analysis environment, allowing data scientists and engineers to experiment with different data sets and models.
Integration
Data lakes can integrate data from multiple sources, including cloud data warehouses and traditional analytics/BI systems. This integration capability makes them a versatile choice for organisations looking to consolidate their data assets.
Advantages Of Data Lakes
Flexibility
Scalability
Advanced Analytics
DATA WAREHOUSE
Understanding Data Warehouses
Definition And Purpose
A data warehouse is a centralised repository that stores large volumes of structured data for querying and analysis. Typically used for business intelligence (BI) and reporting, data warehouses are optimised for complex queries and high-performance read operations.
Data Types And Storage
Data warehouses store structured data that has been:
- cleaned,
- transformed and
- organised into schemas, such as star or snowflake schemas.
They typically use ETL (Extract, Transform, Load) processes to integrate data from various sources.
Use Cases
Data warehouses are ideal for operational reporting, historical data analysis, and business intelligence. They provide a stable and efficient environment for running complex queries and generating reports.
Integration
Data warehouses often integrate data from transactional systems, relational databases, and other structured data sources. This integration ensures that the data is consistent, reliable, and ready for analysis.
DIFFERENTIATORS
Key Differences Between Data Lakes and
Data Warehouses
Data Structure
Data Lakes: Store raw, unprocessed data in its native format, allowing for schema-on-read.
Data Warehouses: Store processed and structured data, requiring schema-on-write.
Schema
Data Lakes: Follow a schema-on-read approach, enabling flexibility in data processing.
Data Warehouses: Use a schema-on-write approach, requiring data to be structured before loading.
Use Cases
Performance
Cost
Data Lakes: Can be more cost-effective for storing large volumes of diverse data.
Data Warehouses: This may incur higher costs due to the need for data processing and structuring.
INSIGHTS
Actionable Insights for IT Leaders, Data Scientists and BI Professionals
-1.png)
For IT Leaders
- Evaluate Your Data Strategy: Assess your organisation's data needs and determine whether a data lake, data warehouse, or combination is the best fit. Consider factors such as data volume, variety, and use cases.
- Plan for Integration: Ensure seamless integration of data lakes and data warehouses with your existing IT infrastructure to enable you to leverage the strengths of both solutions.
- Invest in Skills and Training: Equip your team with the necessary skills to manage and optimise data lakes and data warehouses. Training in data management, cloud technologies, and analytics tools is essential.
For Data Scientists
- Leverage Data Lakes for Advanced Analytics: Utilize data lakes to store and process diverse data sets for machine learning and data science projects. The flexibility of data lakes allows you to experiment with different models and techniques.
- Focus on Data Quality: Ensure that the data stored in data lakes is well-curated and annotated to improve the accuracy and reliability of your machine learning models.
- Collaborate with IT Teams: Work closely with IT teams to ensure the data infrastructure supports your analytics needs. This collaboration will help in optimising data storage and processing.
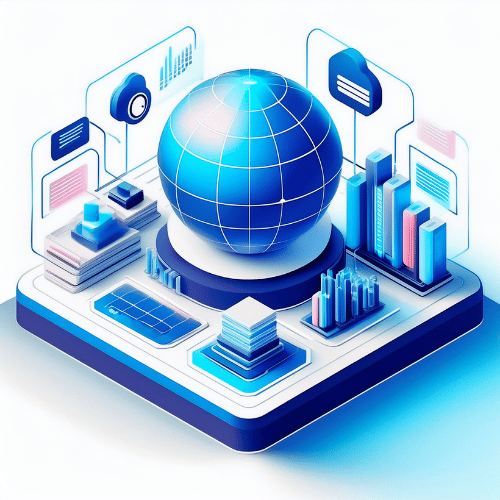
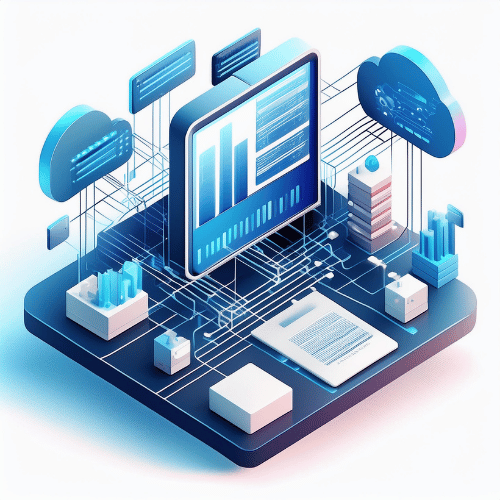
For BI Professionals
- Optimise Data Warehouses for Reporting: Use data warehouses to store structured data and run complex business intelligence and reporting queries. Ensure that the data is consistent and reliable.
- Enhance Data Visualization: Invest in advanced BI tools and techniques to visualize data effectively to help generate actionable insights and improve decision-making.
- Integrate with Data Lakes: Consider integrating data warehouses with data lakes to leverage the strengths of both solutions. This integration will enable you to access a broader range of data for analysis.
SUMMARY
1.
2.
3.
INTERESTED IN LEARNING MORE?
Book a Free 30-Minute Consultation
Your business' data has potential and it can reach new heights with a Free 30-Minute Consultation from Analytium! Book a meeting with our expert, Sander De Hoogh, and get personalised insights into optimising your data strategies.
Thank you for considering Analytium. We look forward to helping you achieve your data-driven goals. Click below to schedule your consultation and start transforming your data.
During The Call, You Can Expect:
- A brief analysis of your current data challenges
- Recommendations tailored to your business needs
- An overview of how Analytium’s solutions can drive your success

July 17, 2024